
Machine learning has been popular since 2012, and AI is now in full swing. Machine learning is an applicable solution of computer vision. When the car camera uses AI, the technical requirements for CIS will also change. Can traditional CIS still take on the 'heavy responsibility'
'Car camera' itself is not a clear word, because today's cars carry more and more cameras, and the specifications and standards of cameras with different functions are different. Yole Development has roughly divided the on-board cameras into ADAS cameras, visual cameras (such as look around, front view, blind spot monitoring, etc.), cameras in the cabin (such as driver monitoring, gesture UI, presence detection, etc.), CMS (Camera Mirror System), and AV autopilot (front view, look around cameras, etc.).
This classification seems to overlap, but there are differences between the cameras inside and outside the car in the general direction, and there are differences between the cameras for people to see and those purely used for data analysis. For example, the photos taken by the mobile phone camera are required to be good looking, and they should be sent to the friends circle; The car camera should take 'capture' as the highest criterion.
The camera on the car can also be divided into two categories according to whether it is visible to people. At the beginning of the article, it is mentioned that the visual camera, namely, the visual application, needs to be shown to people, such as the electronic rear-view mirror and some look around systems. Another type is computer vision (machine vision), which is applied to automatic driving and parking. The value of the pictures taken by this camera is not to show people, but to do data analysis.

This paper mainly discusses the latter type of vehicle cameras (and cameras related to intelligent transportation topics). ADAS and many cameras in the automatic driving direction belong to this category. In this case, image processing for the purpose of human visual friendliness is of no value - computer vision (CV) means that, with images or videos as input, the output is attribute information such as distance, color, shape, object classification, semantic segmentation, etc. What computer vision hopes to achieve is to understand the world you see (although current computational photography actually has some of these attributes).
In the field of computer vision, AI has become a topic that has to be talked about: computer vision can achieve faster and more accurate image classification, object detection, object tracking, semantic segmentation, etc.
When the on-board cameras (mainly ADAS and AV related cameras) are applied to computer vision, what new development directions and technical requirements do image sensors have. We know that computer vision is now so closely related to AI. From a different perspective, it can also be understood as what new developments have taken place in image sensors when car cameras adopt AI technology.
Strict image quality requirements for vehicle specification level
Strict image quality requirements for vehicle specification level
What are the technical requirements for on-board image sensors?
It is not realistic to list all the test items mentioned in the standards and specifications. Therefore, we try to summarize the requirements and new development directions of ADAS/AV machine vision for image sensors according to the current promotion points of image sensor enterprises and the new part of the new standard for vehicle cameras. Here we only discuss the image quality, not the strict requirements of the vehicle specification level itself on the temperature, weather and other aspects of electronic components.
In 2018, Smithers wrote a report entitled autonomous vehicle Image Sensors to 2023 – a State of the Art Report. In addition to the fact that in the future, fully automatic driving will become more dependent on machine vision, and the image enhancement requirements for human vision will become lower and lower, several trends are also mentioned:
(1) It has a dynamic range of 140dB, a monocular resolution of 8 million pixels, a stereo vision camera of 2 million pixels, a frame rate of more than 60fps, and high sensitivity;
(2) Under 0.001 lux illuminance, the signal to noise ratio is greater than 1 when the exposure time is not greater than 1/30 seconds;
(3) While HDR has a high dynamic range, it requires less motion artifacts;
(4) The LED flicking problem is eliminated.
These points are not unexpected, and they are also the direction that contemporary image sensor manufacturers are generally striving for, including the high dynamic range, higher resolution, frame rate of the image sensor itself, as well as the sensitivity and low noise under low illumination. Although some of the parameters listed in the report are ahead of schedule, current image sensor manufacturers have their own trumpets in achieving these goals. For example, for low light environment, many manufacturers are changing the pixel structure, and at the same time, they begin to use dual gain conversion to take into account the daytime and nighttime scenes.
What is worth mentioning here are the last two points mentioned in the trend. First, HDR has a high dynamic range and requires lower motion artifacts. Among them, HDR is the basic feature of the car camera. After all, when the scene light ratio is large, if some areas of the image taken by the camera are overexposed or underexposed, the computer vision analysis cannot obtain the corresponding data. From the perspective of image sensor, HDR can be realized by multiple exposure, double gain, pixel size separation, and improving pixel well capacity.
The HDR scheme of time-domain multiple exposure is very common in the imaging field, but its applicability in the ADAS/AV direction is gradually decreasing - because the vehicle camera requires that the picture should not have motion artifacts, and LED stroboscopic must be suppressed. Therefore, manufacturers like Stevie tend to adopt the single frame spatial domain multi exposure scheme. The typical method is interlaced multi exposure: every two lines of long exposure on the image sensor, and two lines of short exposure. ISSCC has included Stevie's papers on single frame HDR technology.
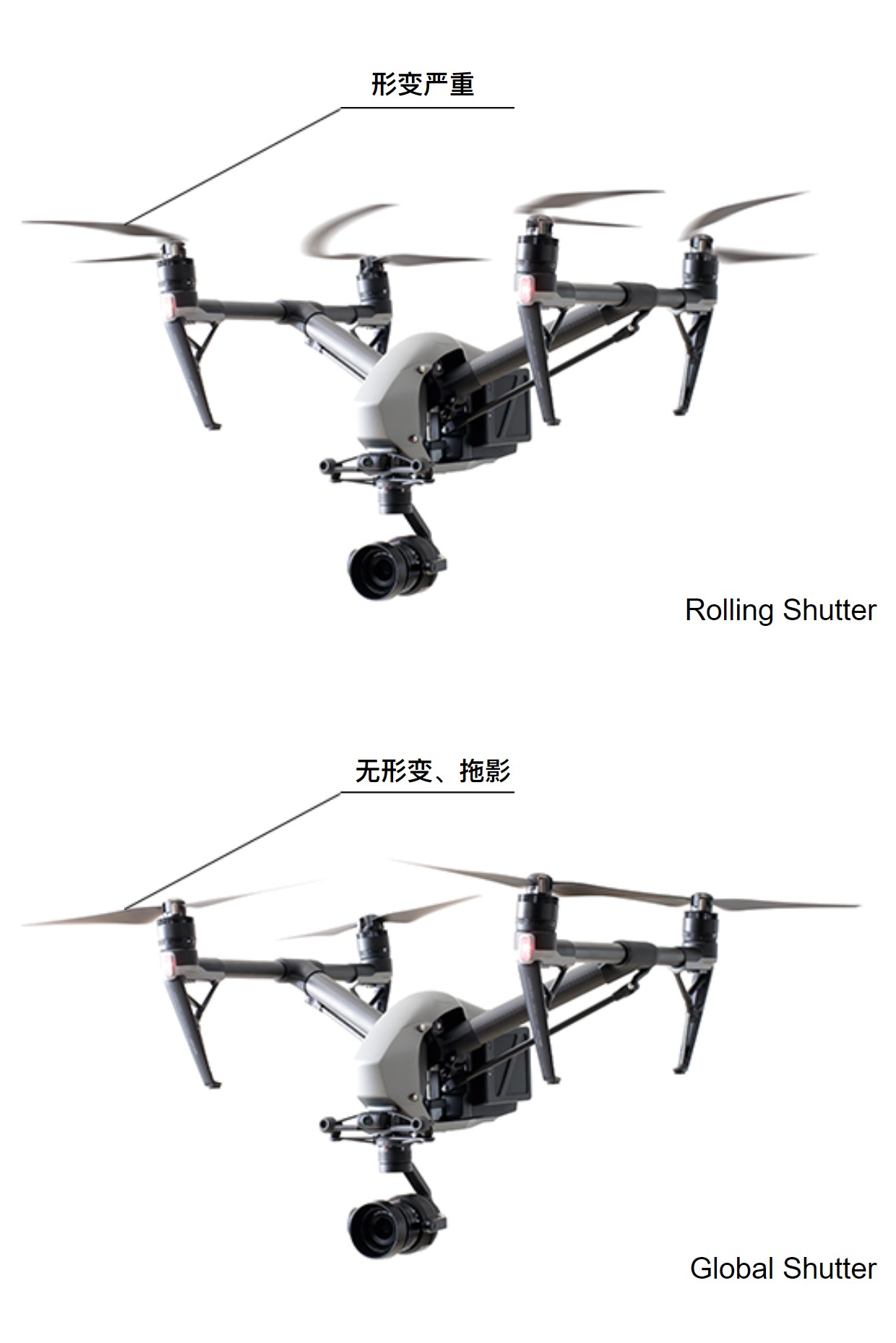
Figure 1, Source: Stevie
In addition, global exposure (or global shutter) is a key point in suppressing motion artifacts. Global exposure is also the technical highland that almost all image sensor manufacturers are fighting for now. Because the traditional rolling shutter uses a progressive exposure method, there will be a jelly effect when shooting high-speed moving objects (Figure 1). Global shutter allows all pixels to start and end exposure at the same time, which avoids this form of image distortion.
Sony's Pregius global shutter sensor is well-known - ISSCC has included a Sony paper that each pixel uses an independent ADC (pixel level interconnection), which is one of the technologies for fast readout to achieve global shutter; OmniVision has a similar technical introduction. The fourth generation global shutter image sensor released by Sony this year finally uses BSI backlight technology to reduce the pixel size. Previously, Stevie also mentioned in its publicity that Stevie is 'one of the few companies in the world that takes the lead in integrating global exposure with BSI technology'.
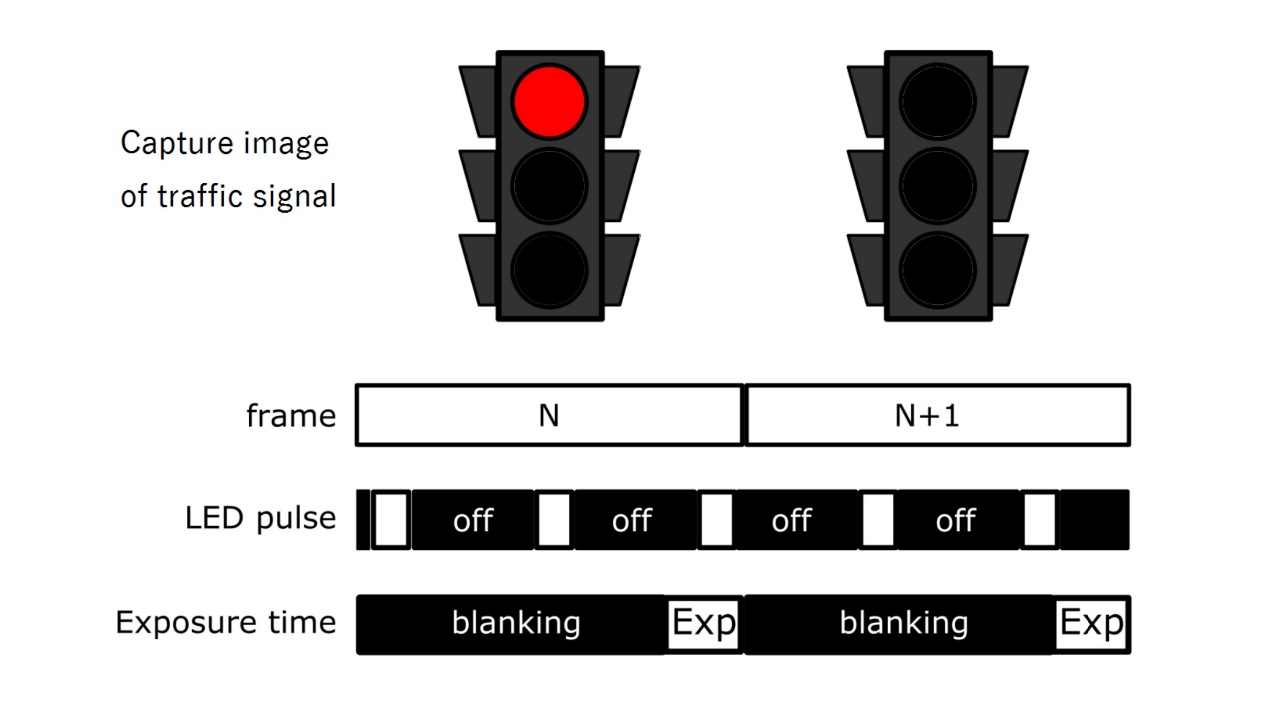
Figure 2, Source:IEEE P2020 Automotive Imaging White Paper
As for the LED stroboscopic elimination mentioned above (Figure 2), in the past two years, Stevie and OmniVision have spared no effort to promote their own image sensor LED flicker suppression technology. After all, in the field of automatic driving and intelligent transportation, LED stroboscopic leads to incomplete second reading information of traffic signs and traffic lights captured by the camera, which will lead to false judgment of the back-end AI system.
In general, LED stroboscopic suppression can be achieved by keeping LED stroboscopic and image sensor shutter synchronized. The problem is that different LED specifications are inconsistent, so this theoretical scheme is not feasible. In addition, HDR can also be used to capture more comprehensive information with a longer exposure time when capturing the picture of LED lights. Image sensor manufacturers apply this big idea, although there are differences in specific implementation. For example, OmniVision applies size separated pixels.
The future trend of machine vision perception has been determined
From a more systematic perspective, there are probably some technical trends worth talking about. For example, from the perspective of sensors, the fusion of various sensors in the vehicle field will be a trend, not only for multi camera systems, but also for the fusion of different types of visual sensors, such as LiDAR and camera fusion sensors; There are also RGB IR sensors, which integrate infrared perception into image sensors (if the scope of sensor discussion is extended to IR sensors, it may also involve near infrared response enhancement and other technical trends, SWIR infrared cameras, etc.).
From the perspective of the entire imaging/vision system, image sensors will integrate some edge computing power, which may also become a trend. For example, Sony has combined AI edge computing with image sensors (IMX500); SK hynix also mentioned in the press release last year that it is feasible to add a simple AI hardware engine to the ISP at the lower layer of the sensor in the stack sensor based on the advanced semiconductor manufacturing process... although these are not yet oriented to the automobile market.
Two years ago, Stevie launched the AISENS sensor chip platform, which is the 'universal AI sensor chip platform integrating perception and computing'. Previously, Stevie also mentioned that the data processing die and sensor die are packaged together in a 3D stacking manner. It seems that this platform is becoming more commercialized.
Last year Yole Development released a report entitled '2019 Market and Technology Trends of Image Signal Processor and Visual Processor'. The report clearly stated: 'AI has completely changed the hardware in the vision system, and has had an impact on the entire industry.' 'Image analysis has added a lot of value. Image sensor vendors are beginning to be interested in integrating the software layer into the system. Now image sensors must go beyond the ability of simply capturing images to analyze images.'
In 2019, EE Times published an article entitled ADAS: Key Trends on 'Perception'. This article discusses the development trend of ADAS vehicle sensing system from a more systematic perspective, and it is recommended to read.
Finally, I would like to briefly talk about the event based visual sensor technology represented by Prophesee. The elite interview of the next Electronic Engineering Album is to interview Luca Verre, CEO of Prophesee, in which this kind of neuromorphological vision technology is introduced in relatively detail. The feature of this sensor is that it is not limited by the frame rate (it can achieve an equivalent frame rate of 1 fps), and only records information when the objects in the scene change dynamically.
Its advantages lie in less data generated, fast response speed and high dynamic range. And this technology is naturally applicable to machine vision. Luca Verre said in an interview: 'In the field of machine vision, we believe that the event based visual sensor can replace the traditional frame based image sensor technology. In the field of imaging, the traditional image sensor technology itself is no problem.' There will probably be a new wave of changes in the field of machine vision.
The original text was published in the International Electronic Business Information sister magazine Electronic Engineering Album, May 2021